Revolutionizing Patient Data Analysis: Boston Medical Center's Journey with Machine Learning
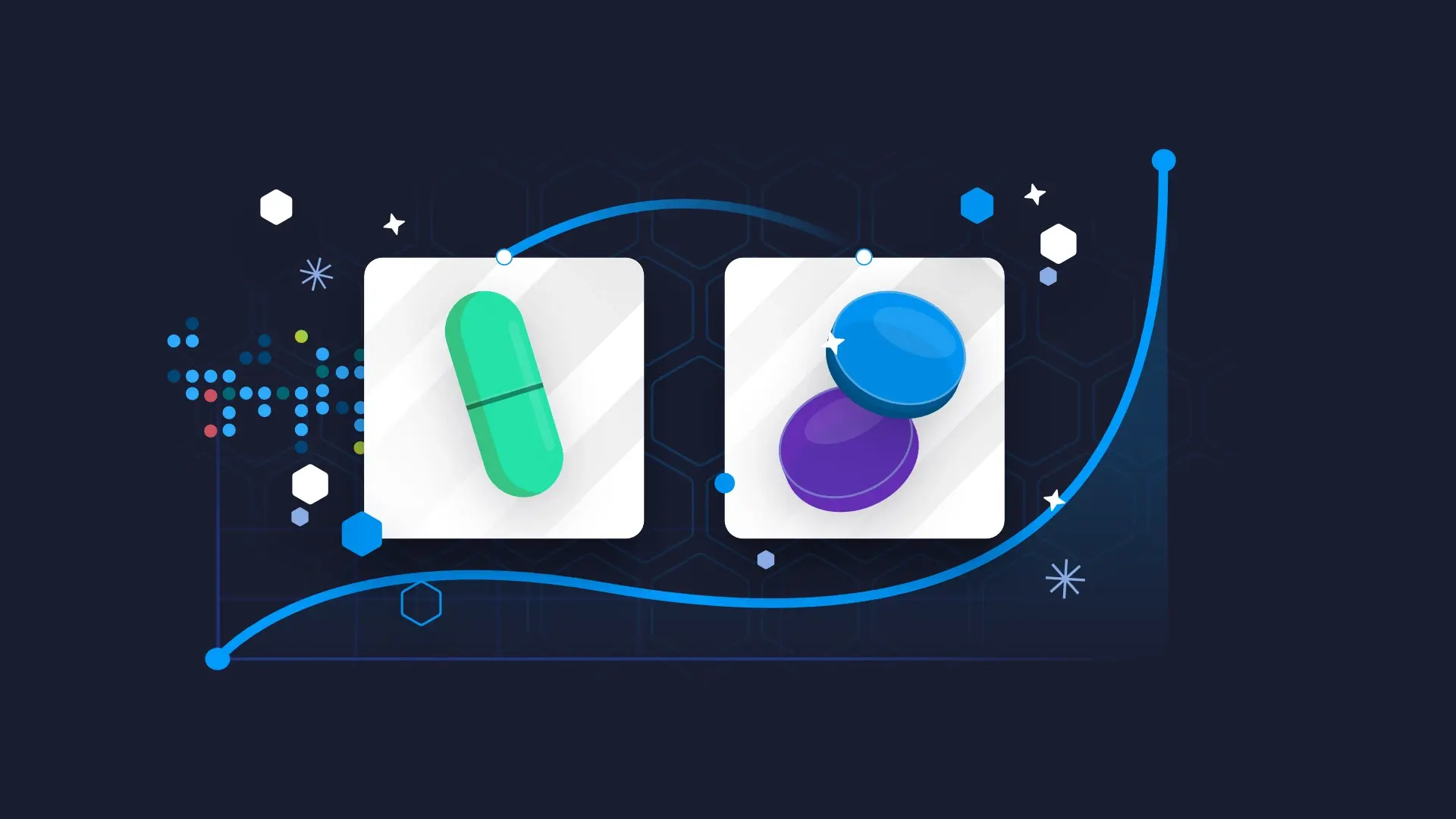
In partnership with the renowned Boston Medical Center (BMC), our Machine Learning team embarked on a mission to <a href="https://www.appsilon.bio/" target="_blank" rel="noopener">revolutionize healthcare data analysis</a>. This collaboration centred around a <strong>powerful Proof-of-Concept (POC) that brings <a href="https://appsilon.com/ai-research/" target="_blank" rel="noopener">Machine Learning (ML)</a> to the forefront of patient data management</strong>.
More than just a tech upgrade, this venture marks a shift in how healthcare professionals approach and utilize patient data.
In this article, we dive into this innovative project, showcasing how ML has <strong>revolutionized patient care and set new benchmarks in healthcare analytics</strong>.
<h3>TL;DR</h3><ul> <li><a href="https://www.bmc.org/" target="_blank" rel="noopener noreferrer">Boston Medical Center (BMC)</a> partnered with our technology team to revolutionize healthcare data analysis using Machine Learning (ML).</li> <li>This project aimed to transform the way patient data is managed and utilized, moving beyond traditional data visualization to comprehensive analytics.</li> <li>The initiative tackled the challenges of <strong>managing large-scale</strong>, <strong>complex patient data</strong> by incorporating societal and environmental factors, using an <strong><a href="https://appsilon.com/r-shiny-pharma-biotech-examples/" target="_blank" rel="noopener">R Shiny app for data visualization</a></strong>, and integrating <strong>advanced ML techniques</strong>.</li> <li>Key ML techniques used included Principal Component Analysis (PCA) for data dimensionality reduction, various clustering methods, and decision tree classifiers for predictive modelling. SHAP values were used for the interpretability of model decisions.</li> <li>The results showed<strong> insightful patient segmentation and predictive analysis</strong>. Also, the project's success demonstrated the potential of data science and ML in enhancing patient care.</li></ul>
<h3>Table of Contents</h3><ul><li><strong><a href="#genesis">The Genesis of a Cutting-Edge Collaboration</a></strong></li><li><strong><a href="#goals">Strategic Goals and Technical Roadmap</a></strong></li><li><strong><a href="#challenges">Navigating Challenges with Advanced Solutions</a></strong></li><li><strong><a href="#deepdive">Technical Deep Dive</a></strong></li><li><strong><a href="#impact">Impact and Future Implications</a></strong></li></ul>
<hr />
<h2 id="genesis">The Genesis of a Cutting-Edge Collaboration</h2>
Boston Medical Center's (BMC) quest for <a href="https://www.appsilon.bio/" target="_blank" rel="noopener">advanced healthcare solutions</a> led to a key partnership with our technology team aimed at revolutionizing patient data analysis. Initially challenged by the limitations of their patient health visualization dashboard, BMC recognized the need for a more sophisticated approach. This led to the strategic integration of advanced Machine Learning (ML) algorithms, moving beyond <a href="https://appsilon.com/accessible-research-with-r-shiny-ui-design/" target="_blank" rel="noopener">data visualization</a> to a more comprehensive analysis.
This collaboration was more than a technological upgrade; <strong>it was a paradigm shift toward data-driven patient care</strong>. By embracing ML, BMC opened new avenues for <strong>personalized healthcare</strong> and optimized patient outcomes, marking the beginning of a transformative journey in healthcare data analytics.
<iframe title="YouTube video player" src="https://www.youtube.com/embed/p1qaUQ0cym8?si=KLg72K51ZpZ5Me2p" width="560" height="315" frameborder="0" allowfullscreen="allowfullscreen"></iframe>
<h2 id="goals">Strategic Goals and Technical Roadmap</h2>
Boston Medical Center (BMC) focused on elevating patient care through advanced data analytics, with a commitment to the OMOP (Observational Medical Outcomes Partnership) Common Data Model for standardized healthcare data. This strategic vision was brought to life through a technical roadmap emphasizing the integration of Machine Learning (ML) algorithms. This approach aimed to transform patient data into actionable insights for improved health outcomes.
Central to BMC's strategy was developing <strong>a scalable and flexible analytics platform, adaptable to the evolving healthcare technology landscape</strong>. This initiative not only serves BMC's immediate needs but also presents a model for other healthcare organizations looking to harness the power of data analytics in patient care.
<blockquote>Experience the future of health-tech. Dive into '<a href="https://appsilon.com/assessing-musculoskeletal-health-with-wearables-and-ai/" target="_blank" rel="noopener">Assessing Musculoskeletal Health with Wearables and AI'</a> to stay learn more.</blockquote>
<h2 id="challenges">Navigating Challenges with Advanced Solutions</h2>
Confronted with the daunting task of managing large-scale patient data, Boston Medical Center recognized the necessity for predictive analytics to draw meaningful insights from their extensive data sets. The challenge was not only in the volume of data but also in its complexity, which required a sophisticated approach beyond traditional data processing techniques.
In response to this challenge, BMC took a multi-faceted approach. This began by emphasizing <strong>integrating societal and environmental factors</strong> into the healthcare datasets, going beyond traditional clinical data. This holistic approach involved incorporating factors such as <strong>geospatial maps, green space, air quality and various environmental aspects</strong>. By assimilating this comprehensive set of data points, BMC’s machine learning model gained enhanced predictive capabilities and a deeper understanding of how different patient features relate to specific health outcomes. This approach not only facilitated the creation of more personalized healthcare solutions but also played a vital role in addressing the broader health determinants that significantly impact patient outcomes.
Additionally, developing an R Shiny app marked a significant step forward, <strong>offering an interactive and flexible platform for real-time data analysis and visualization</strong>. This tool played a crucial role in simplifying complex data, making it accessible and actionable for healthcare decision-makers. To further enhance their analytical capabilities, BMC integrated advanced machine learning techniques into their toolkit.
<blockquote>Unravel the complexity of drug interactions. Delve into '<a href="https://appsilon.com/exploring-drug-interactions-shiny-app/" target="_blank" rel="noopener">Exploring Pharmacokinetic and Pharmacodynamic Drug-Drug Interactions with our Drug Interactions Shiny App</a>' to enrich your understanding.</blockquote>
<h2 id="deepdive">Technical Deep Dive</h2>
Our approach was a blend of sophisticated algorithms and strategic data processing methods designed to extract maximum value from BMC's extensive patient data.
<h3>Algorithms and Techniques:</h3><ul><li><strong>Principal Component Analysis (PCA):</strong> This technique was pivotal in reducing the dimensionality of the dataset while preserving its essential characteristics. By identifying and prioritizing the most informative features, PCA streamlined the analysis process, enabling more efficient data interpretation.</li></ul>
<img class="size-full wp-image-22880" src="https://webflow-prod-assets.s3.amazonaws.com/6525256482c9e9a06c7a9d3c%2F65e9e678a8460f13cd30d9fa_d527ccd9_Principal-Component-Analysis.webp" alt=": A scatter plot of principal component analysis (PCA) for K=5 with a table of selected data points below it." width="727" height="799" />
Principal Component Analysis
<ul><li><strong>Clustering Methods:</strong> We used various clustering techniques for medical data, including K-means for continuous data, K-modes for categorical data, and K-prototypes for mixed data. Users can choose the number of clusters and evaluate results using visualization and relevant metrics for their specific dataset.</li></ul>
<img class="size-full wp-image-22883" src="https://webflow-prod-assets.s3.amazonaws.com/6525256482c9e9a06c7a9d3c%2F65e9e67883f55f13fa14e774_8a4925b8_Clustering-Methods.webp" alt="A line graph depicting the relationship between the number of clusters (K) and the cost of clustering, along with a user interface element to select the K range and a 'Run Clustering' button." width="728" height="844" />
K-Prototypes Clustering Cost Analysis for Varying Number of Clusters
<ul><li><strong>Decision Tree Classifiers:</strong> For predictive modelling, decision trees were utilized. These classifiers helped map out probable outcomes based on a set of decision rules derived from the data features. This approach was particularly effective in identifying key factors influencing patient health outcomes.</li></ul>
<img class="size-full wp-image-22885" src="https://webflow-prod-assets.s3.amazonaws.com/6525256482c9e9a06c7a9d3c%2F65e9e6796266aa46f4021d98_cdfdc143_Decision-Tree-Classifiers.webp" alt="A visual representation of a decision tree classifier with a specified maximum depth, minimum samples split, and minimum samples per leaf, displaying nodes, branches, and class distributions." width="1570" height="833" /> Visualized Decision Tree Classifier with Parameters and Class Distributions[/caption]
<h3>Analytical Strategies:</h3>
<strong>Interpretability with SHAP Values:</strong> To ensure the models’ decisions were transparent and interpretable, SHAP (SHapley Additive exPlanations) values were used. This provided insights into how each feature influenced the model's predictions, making the outcomes more understandable to healthcare professionals.
<img class="size-full wp-image-22887" src="https://webflow-prod-assets.s3.amazonaws.com/6525256482c9e9a06c7a9d3c%2F65e9e679397e62e9fe6c7fb1_263b9231_Interpretability-with-SHAP-Values.webp" alt=" A bar chart showing the average impact on model output magnitude by feature for different classes using mean absolute SHAP values." width="790" height="340" />
Feature Impact Analysis Using SHAP Values for Model Interpretability
<blockquote>Enhance your expertise in model interpretability. Start your journey with '<a href="https://appsilon.com/please-explain-black-box/" target="_blank" rel="noopener">Please, explain. Interpretability of black-box machine learning models</a>' – a must-read article.</blockquote>
<h3>Results</h3>
The application of these advanced ML techniques and data science methodologies resulted in a highly effective analytics framework for BMC with a Proof of Concept (POC).
<video width="100%" height="auto" src="https://wordpress.appsilon.com/wp-content/uploads/2024/01/bmc_poc_demo-2.webm" loop="true" autoplay="true" controls="true"></video>
The PCA and clustering algorithms offered insightful patient segmentation, while decision tree classifiers provided reliable predictive analysis. The combination of these methods with robust data processing and analytical strategies not only met the project’s objectives but also set a new standard in healthcare data analytics.
<h2 id="impact">Impact and Future Implications</h2>
<h3>Impact</h3>
This project transformed BMC's approach to patient care. <strong>The delivered high-quality POC empowers them to cluster patients into groups and gain deeper insights into the underlying characteristics of each group</strong>. It also enables them to utilize their patient data for predictive analysis of variables of interest as well as tracking and monitoring patient trajectories. This continuous monitoring is key to understanding the effectiveness of treatments and improving healthcare strategies over time, boosting personalized care.
A cornerstone of this project is its commitment to combating systemic racism in healthcare. <strong>By tailoring machine learning algorithms to recognise and address racial disparities, the initiative paves the way for more equitable treatment and outcomes</strong>.
<h3>Future Implications</h3>
The success of BMC’s ML initiative holds substantial promise for the broader healthcare industry. It demonstrates the potential of data science and ML in enhancing patient care, serving as a model for other healthcare providers. This case study highlights the adaptability of such techniques, suggesting their applicability in various healthcare settings for similar transformative results.
In summary, the ML POC at BMC not only achieved significant results in patient data analysis but also set a precedent for the future use of advanced data science in healthcare.
<blockquote>Join us in embracing the power of machine learning to shape a healthier future. <a href="https://appsilon.com/#contact" target="_blank" rel="noopener">Contact us today and let's innovate together</a>.</blockquote>
Contact us!
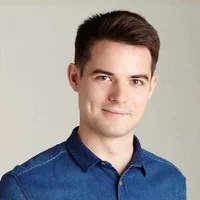